Mathematical models based on characteristics defined by clinical experts are used to educate our AI to best clinically describe the physical reality and emulate clinical reasoning and intuition. When the sensitivity-specificity trade-off glass ceiling is reached, the model’s errors and edge cases become valuable insights for refining these models with expert input, until reaching the boundaries between classes. By re-educating the AI with these new insights—without altering its existing knowledge—it is iteratively improved, ultimately breaking the traditional sensitivity-specificity trade-off paradigm.
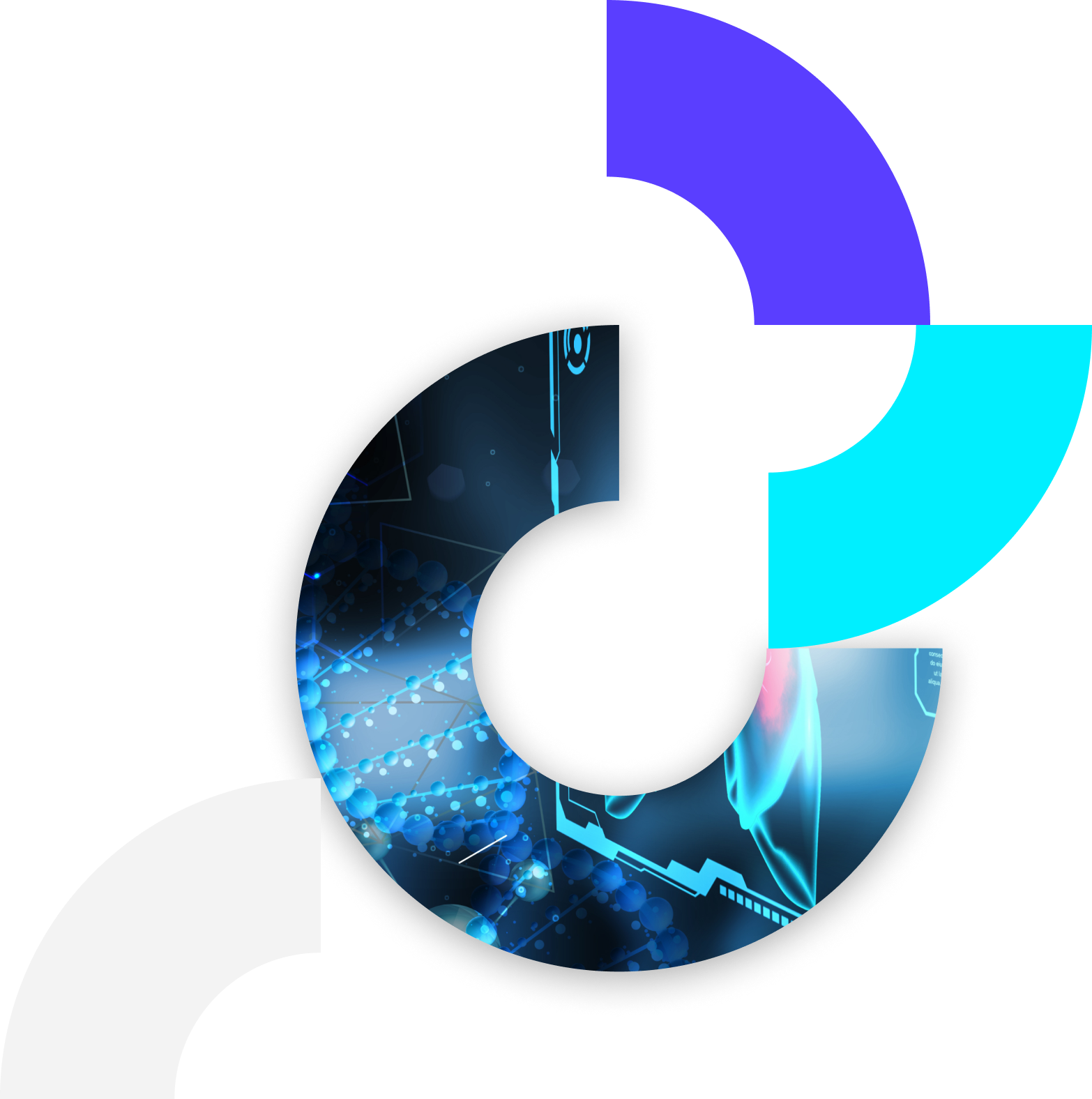
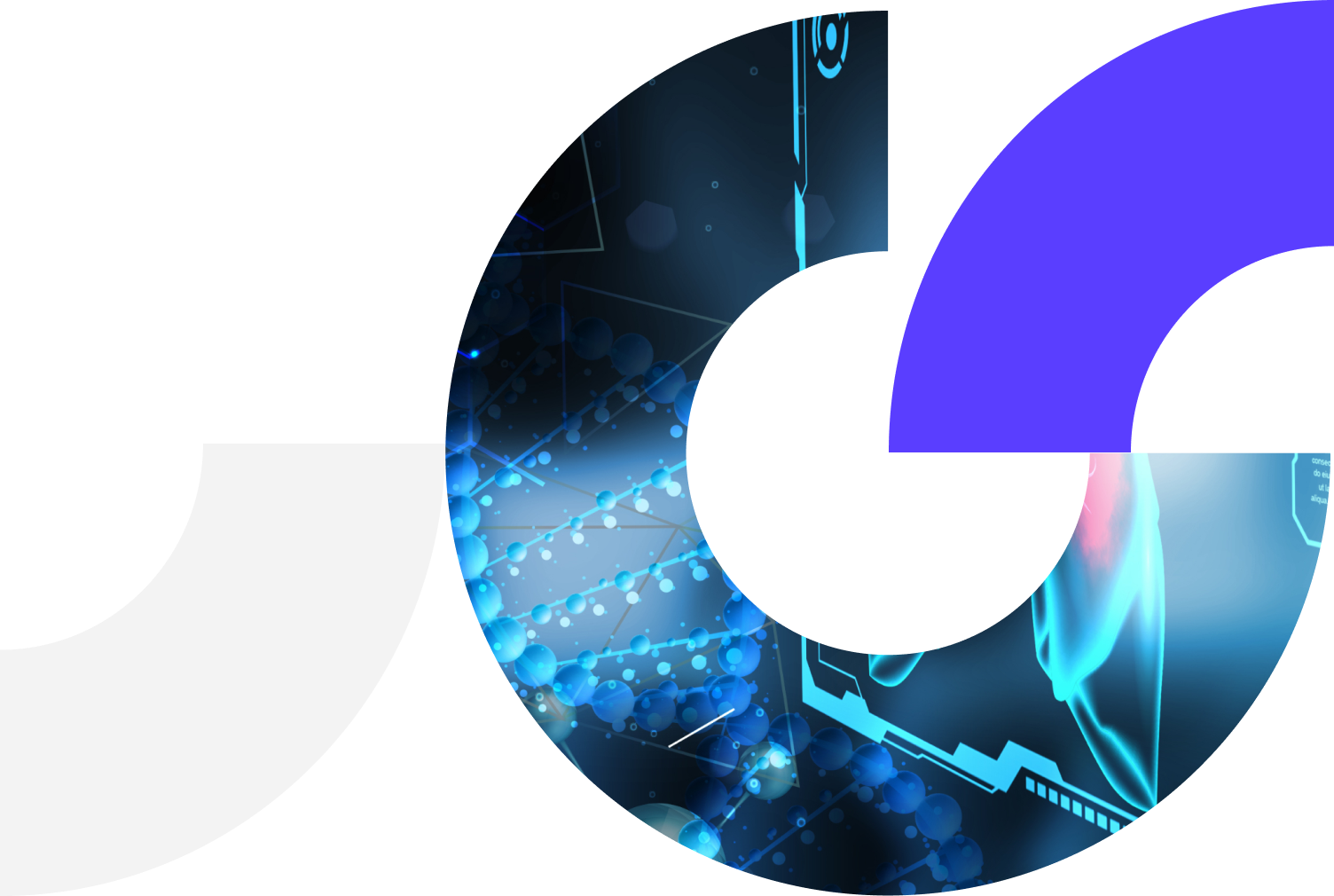
The Clinician’s Co-Pilot for Early Cancer Detection
An agile platform providing timely and reliable insights across multiple cancer types
Learn MoreA non-statistical approach to AI that breaks the sensitivity/specificity tradeoff paradigm
Expert-based mathematical models
Educated AI algorithms
Error detection and edge cases identification
See it in Action
G4Lungs, the first product derived from our platform, is a clinical decision support tool that enables automated lung cancer screening.
Validated on +2,000 real cases from LIDC & Israeli hospitals databases
and in the Assuta clinical study
A new class of AI
Clinically educated to emulate the expert thought process
Explainable logic
that presents findings just as the expert analysis
Minimum data
for high accuracy with near-zero false positives
Minimum data bias
Fast transition from retrospective to prospective use
Indifferent to frequencies and weights
edge cases are modeled just as prevalent ones
Leverage false positives
to improve accuracy
Require only a small amount of data
to new indications by utilizing cross-indication models
Our Pipeline
Lungs cancer
Prostate cancer
Colon cancer
Breast cancer
Ovarian cancer
Brain cancer